Dobb·E
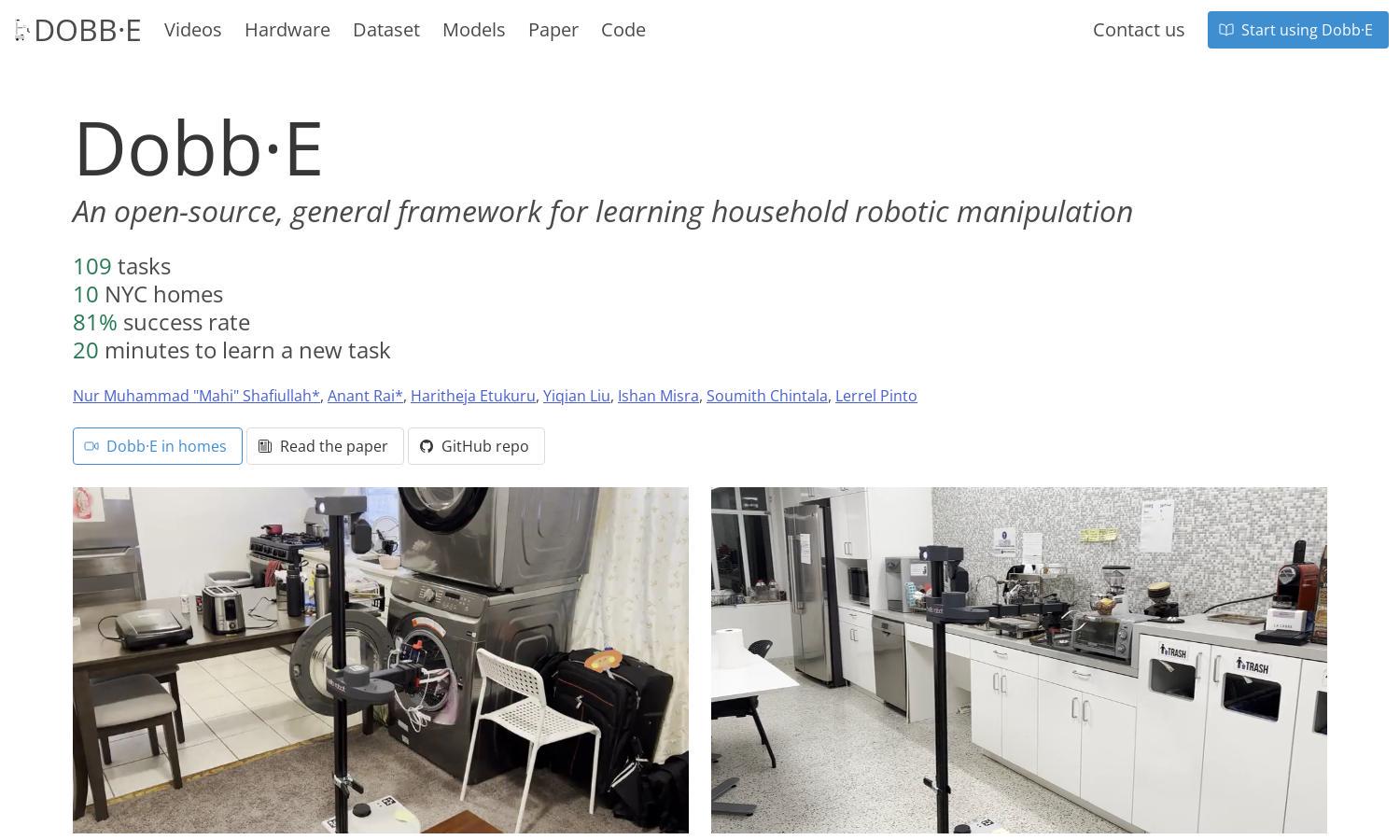
About Dobb·E
Dobb·E is an open-source framework designed for teaching robots to perform household tasks effectively. By utilizing imitation learning and the innovative Stick tool, users can demonstrate tasks in just five minutes, allowing Dobb·E to adapt and learn quickly. This system bridges the gap in home robotics.
Dobb·E offers free access to its open-source framework, and additional premium features may be available soon. The core functionality is free for all users, promoting widespread accessibility to cutting-edge robot learning technology. Upgrading could provide users with advanced support or exclusive features as they develop.
Dobb·E features a user-friendly interface designed for easy navigation and seamless task demonstration. The layout ensures a smooth experience while showcasing the innovative Stick tool and learning models. Users can efficiently interact and train robots, reflecting Dobb·E's commitment to accessibility and functionality.
How Dobb·E works
Users begin with Dobb·E by onboarding through simple instructions showcasing the Stick tool, which is used to demonstrate household tasks. After five minutes of demonstration, Dobb·E captures the data, enabling its representation learning model to quickly adapt and learn the new task within 15 minutes—optimizing the user experience and facilitating effective robot training.
Key Features for Dobb·E
Imitation Learning
Dobb·E's unique imitation learning feature allows robots to learn tasks by observing user demonstrations. This innovative approach reduces training time to 20 minutes, ensuring quick adaptability. By leveraging the Stick tool, Dobb·E simplifies and accelerates the process of teaching robots to handle various household activities.
Homes of New York Dataset
The Homes of New York dataset, pivotal to Dobb·E, comprises 13 hours of diverse household interactions. By offering valuable insights into real-world environments, this dataset enhances the training of robot models, ensuring they perform effectively across various household tasks, elevating the user experience with Dobb·E.
Home Pretrained Representations (HPR)
Dobb·E utilizes Home Pretrained Representations (HPR), a model developed from the HoNY dataset. HPR significantly improves training efficiency and task performance, as it initializes robot policies for varied tasks in new environments. This advanced model exemplifies Dobb·E's commitment to creating adaptable and capable home robots.
You may also like:
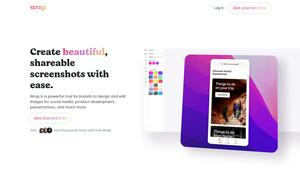